Research
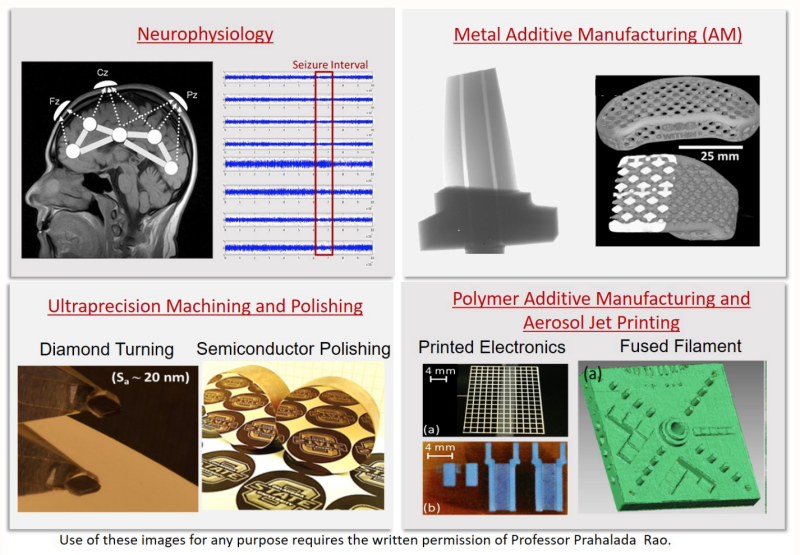
RESEARCH FOCUS
Monitoring and Modeling of Additive Manufacturing Processes.
Understanding and monitoring the root cause of defect formation in Laser Powder Bed Fusion (LPBF), Directed Energy Deposition (DED), Aerosol Jet Printing (AJP), Fused Freeform Fabrication (FFF), and Binder Jetting (BJ).
Read more in-depth articles about these research areas here:
Additive manufacturing (AM), commonly called 3D printing (which is highly irritating to the expert), is a collective term for processes wherein a part is built through layer-upon-layer deposition of material, as opposed to subtractive (machining) or formative (casting, forging, molding) processes.
This technology promises to revolutionize the reach and possibility of manufacturing. For instance, Hod Lipson’s book, Fabricated makes the case for AM as a disruptive technology in terms of 10 different principles; a deeper examination of these principles behooves the following freedoms to AM from the constrains of traditional manufacturing.
- Freedom of infinite complexity – it takes the same effort, for instance, to make a square hole versus a round hole. Indeed, there is no need to restrict to simple Euclidean shapes to ease manufacturing.
- Freedom from assembly – the number of component parts can be reduced by several orders of magnitude. For example, the oft-cited General Electric LEAP engine nozzle has zero sub-assemblies, compared to over 20 parts in the earlier design, while being 25% lighter.
- Freedom of variation in size – the manual effort to scale a part is negligible as no new fixtures need to be made if the size of the part is decreased or increased.
- Freedom from material constraints – there is significantly reduced burden on determining the recipe for different materials, a part can be made from stainless steel or titanium by changing a few parameters (laser power, scan speed, etc.,) as opposed to perhaps changing the tooling.
- Freedom from expensive, skilled labor and experience-driven knowledge – AM processes run almost entirely on their own irrespective of the material and shape being produced.
- Freedom from waste of energy and materials – the amount of material and energy needed is magnitude smaller, for instance, the buy-to-fly ratio in AM, viz., the ratio of material processed to the final weight of the part is as small as 7:1 compared to 20:1 with traditional machining [81-83].
- Freedom from specialized tooling, fixtures, and waste from wear – there is no special tooling or different type of die or tooling required. Because, AM does not require the tool to be harder than the material of the part to be produced there is no wear or possibility of stoppage due to broken dies, etc.
- Freedom from waste of movement and logistics – All parts required for a product can conceivably be made under the same roof, without having to source from different suppliers.
- Freedom of production flexibility, and breaking of batch size scheduling constraints and inventory – the batch size and product mix can be varied at will to match to demand. Hence, given adequate resources, the production rate can be made to match the takt time with zero buffer inventory needed. Furthermore, in a AM-oriented facility, because all machines are identical, there are no bottleneck machines (in the parlance of theory of constraints [84]) that dictate production. If a machine breaks down, there are other identical machine to take the load without having to adjust production.
- Freedom from variability due to breakdowns and maintenance – Because there is variability due to tooling, controlling the input material is sufficient to reduce variability. Furthermore, the uniformity of machines regardless of part design has a great impact on the reliability and maintainability aspects of the production line. Instead of maintaining several service parts and employing repair technicians for various types of machines, a small group of personnel and replacement items is sufficient to keep production at pace.
These freedoms afforded by AM, make it an apt vanguard technology to implement the emerging paradigm of smart and digital manufacturing (Industrie 4.0) as pointed out in the 2011 Whitehouse report on advanced manufacturing. Despite these revolutionary possibilities, recent roadmap reports by federal agencies and national labs emphasize the need for fundamental research in the following areas in additive manufacturing:
- Advanced and novel materials customized to the application.
- Process development to increase the speed and volume of the part produced.
- Design rules and support structure optimization.
- Process modeling and simulations to understand the process physics.
- Predicting the properties of the part, such as its microstructure and geometry.
- Development of in-situ sensing modalities that can capture process and part flaws.
- Algorithms to relate the sensor data to part flaws (in-process quality monitoring and diagnostics)
- Non-destructive evaluation and post-process quality assurance.
- Post-process finishing to improve surface and geometric integrity of free-form surfaces and aid in the removal of supports.
- Standardization of test procedures, geometric dimensioning and tolerancing (GD&T) and metrology of AM parts, standardization of best practices, such as post-process cleaning, and safety benchmarks for handling powders.
- Logistics and supply chain implications of AM.
- Cyber security to defend against intrusion of the digital thread in AM, and protection of design intellectual property.
Answer - Qualify-as-you-build paradigm for AM quality assurace
The LAMPS laboratory research is related to points 3-7 highlighted above. From a technical vista, the current lack of fundamental understanding of the causal process mechanisms, the microstructure of the part produced, and its relationship with the property of the part, i.e., the process-structure-property relationship is at the heart of the current repeatability and reliability-related challenges in AM.
The importance of precision in mission-critical applications, such as aerospace and defense cannot be overemphasized. In a famous paper McKeown, gives the case of how form and finish can almost exponentially increase the effeciency of aerospace compressors. This diagram is remade and produced below.

Unlike machining and forming operations, wherein the surface and near-surface is of concern, and the bulk of the part can be assumed to remain unchanged, in AM the mechanics of surface generation is contingent on each individual layer. A defect at any layer if not detected and averted before the next layer is deposited, is liable to be permanently sealed in, and thus affect the property of the part.
A solution to overcome these quality-related impediments in AM is to qualify the integrity of the part in-process using in-situ sensor data. For instance, SigmaLabs has recently trademarked the phrase in-process quality assurance (IPQA). In a 2013 NSF workshop in AM, Huang et al. suggest the term, certify-as-you-build, wherein the integrity of the part is ascertained as it is being built via the sensor signatures patterns. A slight caveat to the phrase certify-as-you-build is the use of the word certify; certify typically relates to a third party involvement. A slightly different term qualify-as-you-build can be used instead to skirt the unsuitable connotations associated with the former.
Given the lack of consistency of AM processes, industries such as aerospace and biomedical therefore demand extensive post-process inspection of AM parts, such as X-Ray computed tomography (XCT). This is cumbersome and expensive, and will inhibit the drive to incorporate the use of AM for mission-critical parts. This challenge is further compounded by the need for materials testing and process databases to qualify parts for critical components, particularly, for aerospace applications. Because, the sample sizes available in AM are small due to the relatively slow and oftentimes highly specialized nature of the part design, manufacturers therefore do not have the advantage of material performance datasets as in machining and forging which have been developed over the last several decades.
For instance, Gorelik et al. cite the example of a crash of a F/A-18 aircraft in 1981 that was attributed to the premature and catastrophic failure of a turbine disk made using a powder metallurgy process. This accident led to a 5-year decline in powder metallurgy components in the defense industry. Given that AM processes favored for metal parts, such as powder bed fusion (PBF) and directed energy deposition (DED) rely on powder raw material, there is hesitancy on the part of defense manufacturers to adapt AM processes for mission-critical components.
The crux of the challenge towards realizing the qualify-as-you-build paradigm is to establish sensor signature-based process maps, wherein specific sensor signatures are intimately tied to specific types of defects. To build these sensor signature-based process maps requires fundamental understanding of each link in the following AM process chain.
Process Conditions → Process Phenomena → Process Signatures → Part Microstructure (Defects) → Process Control (Rectification) → Part Performance.
Each of these aspects is detailed below.
- Process conditions (e.g., parameter settings, material contamination, part design, machine errors).
- Process phenomena (e.g., vaporization, incomplete fusion, meltpool instability, thermal gradients).
- Sensor data signatures (e.g., meltpool thermal profile, meltpool shape, spatter pattern) extracted from heterogeneous in-process sensors (e.g., thermal and high-speed cameras, photodetectors).
- Part microstructure/defects (e.g., pinhole pores, acicular pores, distortion) caused by the above phenomena.
- Process control (e.g., changing the process parameters, scan strategy, part design, and support structures to compensate for defects)
- Part performance or quality, such as the fatigue life and surface finish.
The progress towards sensor-signature based process maps can be put in perspective in terms of four phases, with each phase often overlapping with the others. We note that none of the following phases can be considered to have been finished; they continue to remain avenues for active research, given the pace at which new materials, processes, and applications are being introduced in AM. Furthermore, each AM process is different, we have to rinse-and-repeat again and again for every new material and process that comes out (which means more work and job security for us at LAMPS!)

Phase 1: Process Conditions → Process Phenomena
In the earlier stages of AM, the emphasis was to establish the link between the process conditions and process phenomena through empirical process variable-based maps for AM processes [94-96]. For instance, in the context of L-PBF this meant relating the areal energy density (EA) in terms of the laser power, scan velocity, and hatch spacing to the structure of the meltpool. To explain further, an inordinately high energy density leads to a phenomena called balling, wherein the meltpool separates into discrete parts due to changes in the surface tension and wetting characteristics [97]. Concomitantly, the laser can enter the so-called keyhole mode, and instead of fusing the powder particles it may vaporize material, leading to pinhole-shaped spherical pores, called gas porosity in the range of 10 µm. In contrast, at low EA the energy required to fuse the powder particles is inadequate, and the material fails to consolidate properly, leading to large (~ 30 µm to 100 µm) acicular porosity.
Phase 2: Process Phenomena → Process Conditions → Part microstructure → Part performance.
Research in this phase seeks to establish the process-structure-property relationship in AM by creating a fundamental understanding of the causal phenomena in AM, and their impact on the functional integrity of the part [98-100]. Staying within this context, the multi-scale nature of AM defects and their dependence on specific process phenomena still remains a significant barrier. For instance, the particle size and laser power used in laser powder bed fusion (L-PBF) is significantly different than blown powder DED. Hence, this phase of process mapping is a tedious and difficult process that involves, process modeling, empirical design of experiments, and finally materials characterization and testing. Each of the links in the process chain summarized above is fraught with challenges.
For example, in L-PBF there are over 50 independent process variables [101]. Investigating the so-called statistical main effects, let alone the interactions, of these variables is cumbersome, and perhaps beyond the scope of traditional design of experiments schemas. Next, the causal phenomena itself occurs at different levels, for instance, in L-PBF the fusion of powder and dynamics of the meltpool has a distinctive effect on porosity. At the higher-scale, the temperature distribution of the part and the heat flux owing to part design is an important aspect influencing the residual stresses in the part. King et al. have used powder-level dynamics and elucidated the effect of laser power, scan speed and hatch spacing on the meltpool shape and splatter pattern [43, 102, 103]. These so-called powder-level models require tracking each particle in and around the meltpool, and require several days to complete on a supercomputer. The higher-level modeling of residual stresses though more tractable, is not easy either, and currently, simulations are reported for a few layers of simple geometries [99].
Phase 3: Process Conditions → Process Phenomena → Process Signatures → Microstructure (Defects) → Part Performance.
The aim of this phase is to capture the process phenomena as they evolve and link them to specific defects through sensor-signatures [86, 90]. This phase is tied to development of new sensor hardware and data analytics to synthesize the sensor signatures into process decisions. Process decisions imply deducing the type, severity, and location of an impending defect based on the sensor signatures. Sensing and monitoring in AM can be stratified per the scale into the following aspects [104]:
- Monitoring of the machine condition, such as the vibration of the recoater, clogging of the nozzle, and the condensation of residue over the optical system.
- Meltpool-level monitoring, such as tracking the temperature distributions and the shape of the meltpool, and relating these characteristics to process conditions.
- Hatch or scan-level monitoring, such as tracking the temperature distribution of each hatch.
- Layer-level monitoring, such as imaging of the integrity of the powder bed using an optical camera or the thermal distribution of the layer.
- Part-level monitoring, such as obtaining the thermal image of the part, or its ultrasonic signature.
These five different sensing strategies are each geared towards detecting a specific type of defect, for instance, meltpool monitoring uncovers porosity in the part, which occurs in the range of 10 µm to 100 µm, whereas, imaging of the powder bed with optical sensors are focused on detecting the onset of delamination and distortion. This detection of multi-scale phenomena mandates heterogeneous sensing, and concomitantly there is a need for efficient methods to synthesize the sensor signatures, and furthermore, to integrate this data with real-time decision-making algorithms. While the sensor hardware is continually improving, the data analytics and decision-making aspect that must be seamlessly integrated with the data acquisition protocol are currently lagging.
Phase 4: Process Conditions → Phenomena → Process Signatures → Microstructure (Defects) → Process Control (Rectification) → Part Performance.
In the final phase, once the type of defect is pinpointed a corrective action must be suggested. The corrective action can take several different forms, including continue to build with changed process parameters or stopping the build to prevent further waste of material. With the advent of hybrid additive manufacturing systems, such as the Maatsura’s Lumex series L-PBF system, DMG Mori-Seiki’s Lasertec DED system, Optomec’s Hybrid LENS system, wherein subtractive machining heads are incorporated within the AM machine, it is possible to entirely remove a layer instead of discarding the print. This new development offers the possibility to extend the qualify-as-you-build concept to a correct-as-you-build paradigm, wherein, the part can be guaranteed to be defect-free. Hybrid AM techniques can therefore be foreseen as akin to an undo command, whereby mistakes in previous layers can be rectified.
At University of Nebraska-Lincoln, LAMPS researchers have access to three such hybrid metal AM machines, and one hybrid polymer AM machine, which puts LAMPS in a position to deliver some pretty awesome and unique capabilities.